The Ultimate Guide to **Image Labeling Tools for Classification**
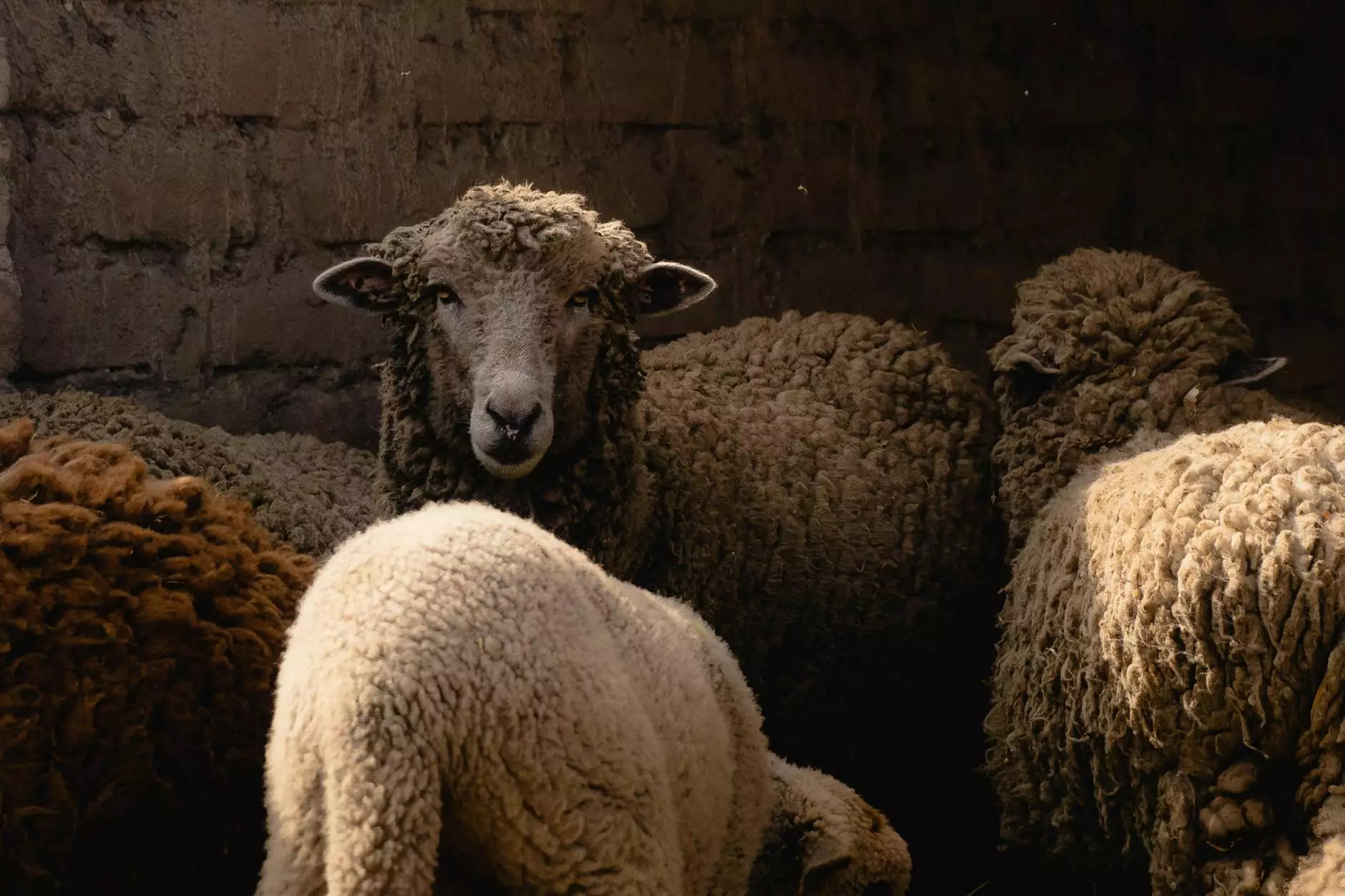
The ever-evolving landscape of artificial intelligence and machine learning has significantly increased the demand for accurate data annotation. One of the most crucial aspects of data preparation in these fields is image labeling, which serves as the foundation for classification tasks. In this article, we will explore the importance of image labeling tools for classification, their features, benefits, and how they can elevate your projects, particularly focusing on the innovative solutions offered by Keylabs.ai.
What is Image Labeling?
Image labeling refers to the process of identifying and tagging specific objects, events, or attributes within images. This process transforms raw images into structured data that machine learning algorithms can understand. The accuracy of these labels is paramount; inaccurate labeling can lead to incorrect model predictions and lower overall performance.
Why Image Labeling is Essential for Classification
In the realm of machine learning, classification tasks involve categorizing data into predefined classes based on its features. Here’s why image labeling is essential:
- Data Quality: High-quality labeled images ensure the dataset reflects the real-world scenarios the model will face.
- Model Performance: Well-labeled datasets improve the accuracy of machine learning models, leading to better predictions.
- Training Efficiency: Structured and labeled data helps in the speedy training of algorithms, reducing time and computational resources.
- Scalability: As data grows, the need for effective labeling strategies becomes even more critical.
Understanding Image Labeling Tools
Image labeling tools are software applications designed to assist users in annotating images efficiently. These tools streamline the labeling process and often come with features that enhance productivity and accuracy. Let's delve deeper into the various types of labeling tools available and their functionalities.
Types of Image Labeling Tools
Various types of image labeling tools cater to different needs and complexities of image data. These include:
- Manual Labeling Tools: Basic tools that require users to tag the images manually. Ideal for small datasets but can be time-consuming.
- Automated Labeling Tools: Utilize AI algorithms to automatically suggest labels based on pre-existing models. These tools can drastically reduce the labeling time.
- Collaborative Annotation Platforms: Allow multiple users to work on labeling tasks simultaneously, increasing throughput for larger projects.
- Cloud-Based Platforms: Offer flexibility and accessibility, enabling users to collaborate and label images from anywhere in the world.
Features to Look for in Image Labeling Tools
When choosing an image labeling tool for classification, several features can make a significant difference in the overall experience and efficiency:
- User-Friendly Interface: A well-designed interface allows users to navigate the tool effortlessly.
- Multi-Format Support: The ability to annotate various image formats ensures compatibility with your existing data.
- Customizable Label Sets: Flexibility to create and manage custom label sets to suit specific project needs.
- Integration Capabilities: Easy integration with machine learning frameworks and other data processing tools.
- Quality Assurance Features: Tools that offer review and validation processes to ensure labeling accuracy.
Benefits of Using Image Labeling Tools for Classification
The advantages of utilizing image labeling tools are numerous. Here are some of the key benefits:
- Increased Productivity: Labeling tools streamline the annotation process, allowing teams to label more images in less time.
- Consistency: Automated tools help maintain consistency across labels, which is vital for model training.
- Cost-Effectiveness: Efficient labeling processes reduce labor costs associated with manual data preparation.
- Enhanced Collaboration: Cloud-based tools enable teams to collaborate remotely, facilitating faster project turnaround times.
- Improved Model Accuracy: High-quality labeled data directly contributes to better-performing models.
Choosing the Right Image Labeling Tool
Selecting the right image labeling tool for classification requires careful consideration of various factors, including your project's scope, your team's size, and your specific annotation requirements. Here’s a guide to help you make an informed decision:
1. Define Your Project Requirements
Understand the type of images you will be working with and the complexity of labeling tasks required. This includes the number of classes, the level of detail in labeling, and the preferred labeling methods.
2. Assess Your Budget
Consider the cost of different tools available and the return on investment you expect. Balance between affordability and the features offered.
3. Evaluate Features
Make a list of essential features that meet your project's needs. Look for capabilities such as automation, collaboration, and integration with other tools.
4. Check User Reviews
Research user feedback to gauge the effectiveness and reliability of the image labeling tools you are considering. User experiences provide valuable insights into the tool's performance.
5. Trial Periods and Demos
Take advantage of trial periods or product demos to test the tools firsthand. This gives you a better understanding of their usability, functionality, and efficiency.
Keylabs.ai: Your Solution for Image Labeling
When it comes to image labeling tools for classification, Keylabs.ai stands out as a comprehensive solution for data annotation. Here’s why:
Advanced Features
Keylabs.ai offers a user-friendly platform equipped with advanced features designed to facilitate efficient image labeling:
- AI-Powered Automation: With intelligent algorithms, Keylabs.ai suggests labels for images, streamlining the annotation process.
- Multi-User Collaboration: Teams can work concurrently on labeling tasks, which enhances productivity and project speed.
- Comprehensive Reporting: Users can track labeling progress and assess data quality through detailed reports.
- Custom Labeling Tools: Adapt the labeling interface and options to fit the unique needs of specific projects.
Scalability
No matter the size of your projects, Keylabs.ai provides scalable solutions that grow with your needs, allowing you to manage small datasets or large volumes of images effortlessly.
Integration with Machine Learning Workflows
Integrate with popular machine learning frameworks, ensuring a seamless transition from data annotation to model training and deployment.
The Future of Image Labeling and Data Annotation
As technology continues to advance, the future of image labeling is promising. The development of image labeling tools for classification is expected to evolve, making the process even more efficient and accurate. Here are some trends we anticipate:
- Increased Automation: More sophisticated AI algorithms will enhance automated labeling capabilities, reducing the need for manual intervention.
- Integration of Augmented Reality: AR technology may play a role in better understanding context during the labeling process.
- Focus on Data Privacy: Tools will increasingly address data protection and privacy concerns, ensuring compliance with regulations.
- Enhanced User Experience: Continuous improvements in user interfaces will make tools more intuitive and accessible to non-technical users.
Conclusion
In conclusion, the role of image labeling tools for classification is undeniably vital in the data annotation landscape. By utilizing a tool like Keylabs.ai, businesses can enhance their data preparation processes, drive machine learning model accuracy, and ultimately achieve better outcomes in their various applications. Whether you're working with small datasets or managing large-scale projects, investing in the right image labeling tool will bridge the gap between raw data and actionable insights.
Embrace the benefits of efficient data annotation and pave the way for innovation in your organization with advanced imaging capabilities today!