Mastering Labeling Training Data for Optimal Business Growth
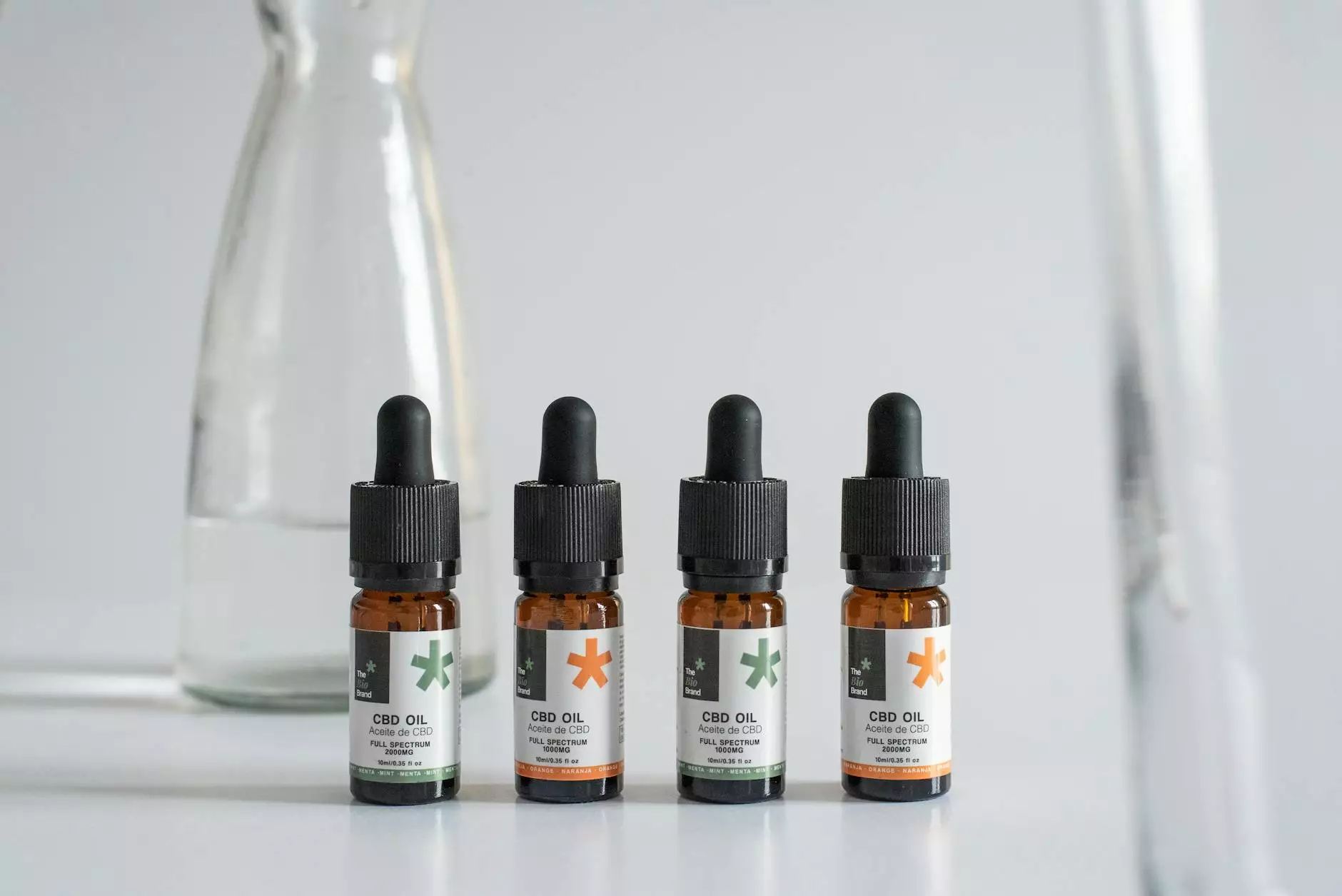
In today’s fast-paced digital landscape, businesses are increasingly dependent on data-driven solutions to drive their strategies forward. One vital aspect of this evolving ecosystem is the labeling training data, a process that plays a crucial role in the development of artificial intelligence (AI) and machine learning (ML) models. Companies like Keylabs.ai provide sophisticated data annotation tools and platforms that can significantly enhance data quality and usability for businesses across various sectors.
Understanding the Importance of Labeling Training Data
Labeling training data involves the meticulous task of tagging and annotating data sets so that machine learning algorithms can learn from them. This process is not just about simple identification; it encompasses an intricate understanding of the data characteristics and intended outcomes.
Why Labeling Training Data Matters
The importance of meticulously labeled training data cannot be overstated. Here are several reasons why:
- Enhanced Model Accuracy: High-quality labeled data ensures that machine learning models can make accurate predictions and decisions.
- Improved Data Quality: Proper labeling helps in cleaning and refining datasets, leading to enhanced data quality overall.
- Time Efficiency: Automating the labeling process reduces the time spent on manual annotations, speeding up the development cycle.
- Accessibility of Insights: Well-labeled data makes it easier to extract actionable insights from complex data sets.
The Process of Labeling Training Data
The process of labeling training data typically encompasses several stages:
1. Data Collection
Before data can be labeled, it must first be collected. This can involve gathering data from various sources, including existing databases, user input, and external datasets.
2. Data Preprocessing
Once the data has been collected, it often requires preprocessing to remove noise and irrelevant information. This step ensures that the data is clean and structured before beginning the labeling process.
3. Labeling/Annotation
This is the core step where human annotators or automated systems tag the data. Depending on the dataset, this may involve categorizing images, transcribing audio, or labeling text.
4. Quality Assurance
Quality assurance measures, such as having multiple annotators review the data or using validation checks, are critical to ensure that the labeled data meets quality standards.
5. Deployment and Monitoring
Once data has been labeled and validated, it is deployed in ML models. Continuous monitoring is crucial to ensure that the model performs well with real-world data.
Choosing the Right Data Annotation Tool
With the importance of labeling training data firmly established, choosing the right data annotation tool becomes paramount. Keylabs.ai offers a comprehensive suite of tools that cater to various annotation needs, making the process more efficient and effective.
Key Features of Keylabs.ai Data Annotation Tools
- User-friendly Interface: A simple and intuitive interface allows users of all skill levels to easily navigate the annotation process.
- Multi-format Support: Supports various data formats, including images, videos, audio files, and text, giving flexibility in projects.
- Real-time Collaboration: Teams can work together in real time, ensuring consistency and rapid project completion.
- Automated Annotation: Leverages AI to assist in the annotation process, thereby increasing productivity and reducing human error.
- Scalable Solutions: Can handle projects of any size, from small datasets to large-scale enterprise-level requirements.
Building an Effective Data Annotation Strategy
Establishing a robust data annotation strategy is essential for businesses leveraging labeling training data. Here are a few steps to consider:
1. Define Objectives
Clearly outline the objectives of your data annotation project. Knowing exactly what you want to achieve will guide every step of the process.
2. Select the Right Tools and Partners
Partner with reliable data annotation tool providers like Keylabs.ai, which offer tailored solutions to meet specific needs.
3. Train Your Team
Ensure that your annotation team is trained on the tools and the specifics of your project. A knowledgeable team can significantly improve the quality of labeled data.
4. Implement Quality Control Measures
Establish metrics and procedures for quality control throughout the annotation process to maintain high standards.
5. Iterative Feedback Loop
Incorporate a system for receiving feedback throughout the labeling process, allowing for adjustments and improvements to be made in real time.
Real-World Applications of Labeled Training Data
The applications of labeled training data are diverse and impactful across various industries. Here are a few notable examples:
Healthcare
In healthcare, annotated data is essential for developing predictive models, such as those used in diagnosing diseases through medical imaging.
Automotive
In the automotive industry, labeled data supports the development of autonomous driving systems, where precise object detection and classification are critical.
Finance
Financial institutions utilize labeled data to detect fraudulent transactions, assess credit risk, and improve customer service through chatbots.
Retail
Retailers label data to enhance customer segmentation, personalize marketing strategies, and optimize inventory management.
Future Trends in Data Annotation
The field of data annotation is evolving, and several trends are shaping its future:
Increased Automation
Tools like those available at Keylabs.ai are increasingly utilizing AI to automate portions of the annotation process, making it faster and more efficient.
Focus on Ethics and Bias Reduction
As AI systems become more integral to business strategies, there’s a growing focus on ethical considerations and reducing biases in training data.
Demand for Custom Solutions
As diverse industries adopt more specialized AI solutions, the demand for custom data annotation services tailored to specific business requirements continues to rise.
Conclusion
In conclusion, the process of labeling training data is vital for the success of machine learning and AI-driven strategies in today’s businesses. Utilizing platforms like Keylabs.ai can significantly streamline this process, enhancing data quality and facilitating better business outcomes. By investing in quality data annotation, businesses are not just staying competitive but are paving the way for future innovations.
To learn more about how Keylabs.ai can transform your data annotation processes, visit Keylabs.ai and explore our advanced data annotation tools and platforms today.